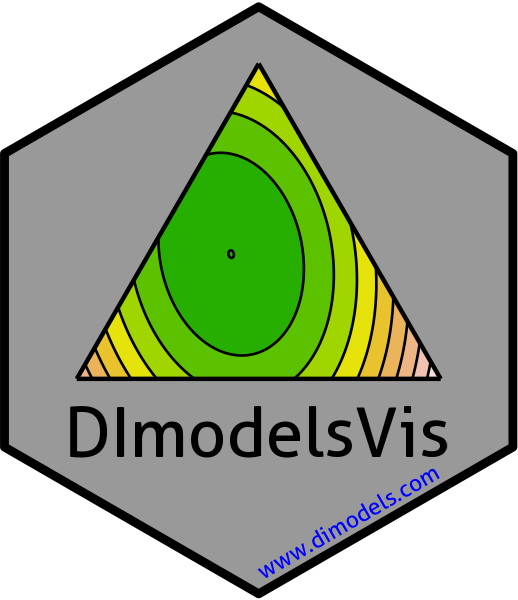
Data preparation for regression diagnostics plots with pie-glyphs
model_diagnostics_data.Rd
This function prepares the data-frame with necessary attributes for creating
regression diagnostics plots for a model with compositional predictors where
points are replaced by pie-glyphs making it easier to track various data points
in the plots. The output data-frame can be passed to
model_diagnostics_plot
to create the visualisation.
Value
The original data used for fitting the model with the response and all model predictors along with the following additional columns
- .hat
Diagonal of the hat matrix.
- .sigma
Estimate of residual standard deviation when corresponding observation is dropped from model.
- .cooksd
The cook's distance (
cooks.distance()
) for each observation.- .fitted
Fitted values of model.
- .resid
The residuals for the observations.
- .stdresid
The standardised (Pearson) residuals for the observations.
- Obs
A unique identifier for each observation.
- Label
The labels to be displayed besides the observations in the plot.
The quantile values for the standardised residuals generated using
qqnorm()
.- weights
The weights for each observation in the model (useful in the context of weighted regression).
Examples
library(DImodels)
## Load data
data(sim1)
## Fit model
mod1 <- lm(response ~ 0 + (p1 + p2 + p3 + p4)^2, data = sim1)
## Get data for diagnostics plot
diagnostics_data <- model_diagnostics_data(mod1,
prop = c("p1", "p2", "p3", "p4"))
print(head(diagnostics_data))
#> response p1 p2 p3 p4 .hat .sigma .cooksd .fitted .resid
#> 1 10.815 0.7 0.1 0.1 0.1 0.07512992 1.343832 0.0005750445 10.47436 0.3406361
#> 2 11.232 0.7 0.1 0.1 0.1 0.07512992 1.340067 0.0028447324 10.47436 0.7576361
#> 3 10.192 0.7 0.1 0.1 0.1 0.07512992 1.344130 0.0003951286 10.47436 -0.2823639
#> 4 8.157 0.7 0.1 0.1 0.1 0.07512992 1.299979 0.0266139030 10.47436 -2.3173639
#> 5 6.724 0.1 0.7 0.1 0.1 0.07512992 1.238479 0.0616767948 10.25177 -3.5277748
#> 6 11.093 0.1 0.7 0.1 0.1 0.07512992 1.338966 0.0035070720 10.25177 0.8412252
#> .stdresid Obs Label .qq
#> 1 0.2660631 1 1 0.06270678
#> 2 0.5917723 2 2 0.45376219
#> 3 -0.2205480 3 3 -0.31863936
#> 4 -1.8100401 4 4 -1.73166440
#> 5 -2.7554644 5 5 -2.39397980
#> 6 0.6570619 6 6 0.54852228
## The compositional predictors in the data are added as attributes to the data
attr(diagnostics_data, "prop")
#> [1] "p1" "p2" "p3" "p4"